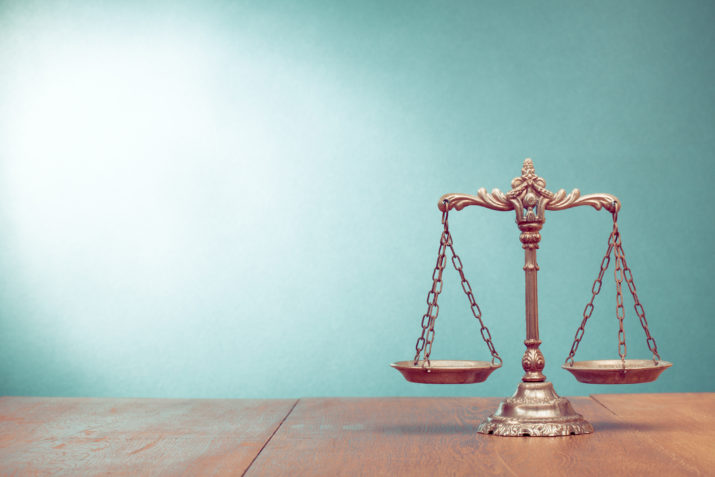
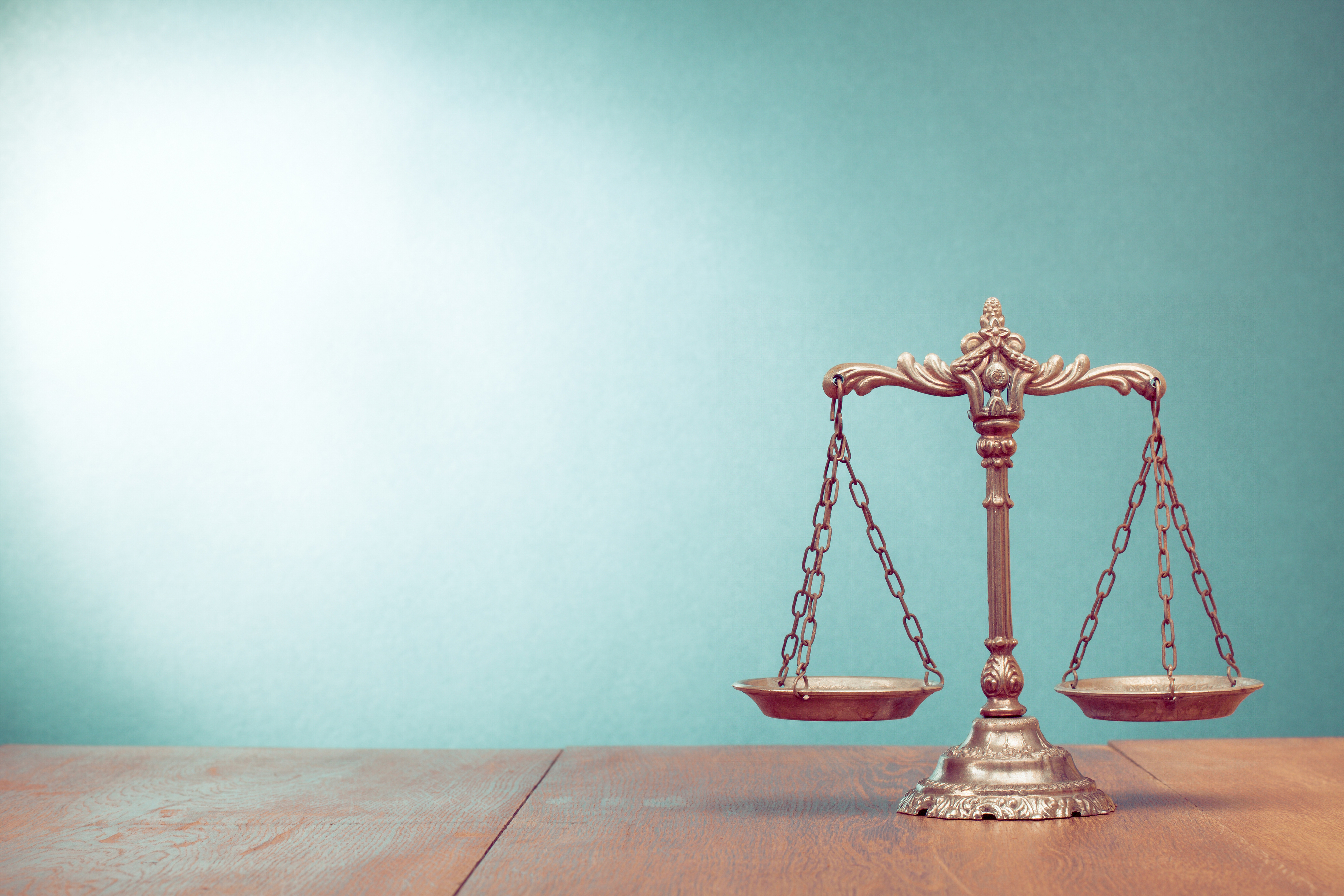
This is part of our special feature on Crime and Punishment.
Decision-makers in the criminal justice system, from judges to parole officers to program eligibility screeners, have long relied on a justice type of triage. Triage is typically used in the medical context to describe the process of rationing medical attention based on the urgency of a patient’s condition.[1] It comes from the French word for sorting or sifting.[2] Its use presumes resources are sparse. In the American criminal justice context, space in a mass incarceration system is presumed. It is space in society that is rationed for people accused of crimes to retain or regain liberty. Triage in the justice system also expedites an otherwise complicated application of new factual circumstances for each accused person, abetting decision-makers in the swift disposal of their dockets at the expense of the attention due to each accused. Antithetical to American constitutional principles, triage in the justice system rations liberty and due process among the accused; it is mainly through legal advocacy that they may fight for their rations.
Naturally, in a system already primed for triage, actuarial risk assessment instruments are spreading rapidly.[3] At nearly every stage of decision-making—including bail, program eligibility, sentencing, probation, prison classification, parole release and supervision—actuarial tools are assisting decision-makers to ration liberty and due process.[4] These instruments usually produce some sort of score for an individual, whether it is a score for their likelihood to return to court, their risk of re-arrest or their risk of failing a program. That score is then relied on either partially or wholly by the decision-maker.
Advocacy in that context requires working knowledge of how an individual’s score is produced and whether it is accurate, yet few advocates have the technical working knowledge of how actuarial decisions, available at various levels of transparency, are generated. Many legal advocates are just now beginning to recognize the potential systemic disadvantages actuarial instruments present to the accused’s ability to claim their rations of justice. Other advocates herald the apparent neutrality and efficiency of the automated decisions as opposed to human decision makers.
This essay will present an introductory overview of discretion involved in developing automated decision tools and their limitations, and discuss how actuarial decision-making instruments definitely change and potentially harm the ability of people accused of crimes to advocate for their justice rations.
Overview of Actuarial Instruments
In the criminal justice system, judges are frequently tasked with making quick and consequential determinations about an individual’s future. For example, in a bail application, judges have to decide whether or not to incarcerate or release someone based on their assumptions about the accused’s likelihood of returning to court or in some states, their likelihood of reoffending.[5] Judges must decide on a threshold at which point the accused’s risks outweigh the cost of incarceration. This decision is often made with mere minutes of deliberation and without sufficient information, time for analysis, or knowledge about what makes one likely to be at risk of absconding or reoffending. It may also be influenced by judges’ own biases or motives that do not have anything to do with reducing incarceration or maintaining public safety. Actuarial instruments would appear to resolve these issues by introducing structure and a basis of knowledge to such analyses. They make predictions based on historical data, evaluate the same factors for each person, and score everyone across the same scale, homogenizing the assessment elements and apparently neutralizing decisions. Despite the portrayed objectivity of risk assessment instruments, they are malleable to subjective decisions at every stage of development.
Packaging Policy as Data Science
The development of actuarial risk assessment instruments is not a purely scientific exercise, but rather a combination of data science and policy decisions. Since data scientists with the expertise to create the models rarely have the specialized or localized knowledge of the context in which they will operate, they rely on input from policymakers and other stakeholders. It is imperative that policymakers and advocates understand the mechanics, limitations, and subjectivity of actuarial instruments.
Variable Selection
The first step in developing an actuarial risk assessment instrument is identifying a measurable outcome to predict. Judges’ generalized concerns over an individual’s risk to public safety must be converted into something observable, such as risk of being arrested for a violent crime. A major limitation of risk assessment, especially in the criminal justice context, is the inability to assess threats that are unobservable or incalculable. Instruments in the criminal justice system almost always use an outcome variable that relies on police crime reporting and arrest data.[6] However, official crime data systematically underreports and over represents certain types of crimes and criminals based on their observability to police.[7] Models cannot detect systematically misrepresentative data if those biases exist in both the training and test data. Naturally, then, the instrument projects the same blind spots and biases.[8]
Along the same lines, the developer must obtain data on measurable factors that correlate with the chosen outcome. While the developer can test whichever potential factors they desire, they are limited by their availability, apparent neutrality, and perceived relevance. Developers are typically constrained to rely on data from police departments, courts, and other government agencies’ whose purpose for data collection has historically been case management. This leaves little room to test for other sociological factors and conditions unrelated to criminal history.
Once an outcome variable and potential predictor variables are identified, the developer must obtain a dataset consisting of the observed outcomes and predictor variables for a sufficiently large sample of the population. The developer then performs some kind of statistical analysis on a portion of the data, which can range in complexity from basic linear regression analysis to unsupervised machine learning. Regardless of the technique, the analysis seeks to identify the correlations between the outcome and the predictor variables.
It is important to recognize that this analysis is limited to identifying the weight of the predictor variables’ effects on the dependent variable rather than causal relationships. Since predictive modeling is a de-contextualized process, seemingly irrelevant or unfair criteria may be highly predictive of the outcome. For example, there is a large association between major felonies and poor air quality in New York City that is likely due to other mediating factors, such as police surveillance of poor, minority neighborhoods.[9] Even though there is no evidence that pollution causes crime, a developer may accordingly include air quality as a predictive factor in a model, ranking those that live in polluted neighborhoods as riskier. In contrast, strong predictive factors might be excluded from a model due to legal objections or fear of public perception.[10] Race, for instance, was often included as a predictor variable in early risk assessment instruments until the 1970s when it was deemed unethical and potentially unconstitutional.[11] This is despite the fact that incorporating a protected characteristic in the model is the best way to control for disparities.[12] Whether or not these choices are fair is a matter of policy rather than data science.
Furthermore, research indicates that decision-makers may not trust an instrument if it does not assess factors that they theoretically or historically believed are relevant.[13] In order to bolster buy-in by judges or other decision-makers, instruments may include factors poorly correlated to the outcome in a model.[14] For instance, the New York City Board of Examiners of Sex Offenders developed an RAI to determine sex offender registration requirements.[15] The Board selected features based on their perceived relevance which resulted in multiple uncorrelated factors being included in the model and many strong predictive factors excluded. Not surprisingly, The New York City Bar found that the instrument “covers and conflates too many disparate concerns with no scientific basis for doing so” and “lacks a coherent methodology and seems to have been designed arbitrarily.”[16] At the end of the day, the developer possesses the discretion to select the features they believe best predict the outcome, adjusting for any objections or suggestions by stakeholders.
Model Selection
Once the relationships between the variables are identified, models are then constructed and tested using another portion of the data. Various performance metrics and requirements are often compared when selecting a model. Since predictive modeling is fixated on optimization, human intervention is required to constrain a model for the contextualized goal of the instrument. The need for simplicity may be more heavily prioritized when constructing a model for use in a courtroom where administrators must obtain all the necessary data and hand-score the defendant in less than ten minutes. A simple model or score sheet that is less accurate may be chosen over a more accurate but more complicated algorithm.
Models may also be calibrated or manipulated in accordance with the developers’ or policymakers’ notions of fairness. For example, if a model consistently ranks black people as riskier because the data shows they have higher rates of re-arrest due to disproportionate police presence in communities of color, then a developer may recalibrate the model to equalize their risk predictions with white people. While there are conflicting debates about what is the fairest model in these circumstances, one thing is clear: these decisions are a matter of policy, not scientific evidence predicting any individual’s future behavior.
Ultimately, a final model is selected and any individual’s data can be put into the model to calculate a prediction. At the most basic level, the model is computing the ratio of people in the sample data with the same factors as the current individual that presented or did not present the outcome; for example 45% of people with the same factors as this individual returned to court for every appearance.[17]
Model Output
The raw output of the model is rarely the statistic that is reported by the instrument. Instead, a risk probability is often condensed into a categorical (low-risk, medium risk, high risk) or ordinal (1-5) risk ranking. None of the five most prominent risk assessment instruments – COMPAS, PSA, PCRA,YLS/CMI, nor LSI-R – report the actual risk likelihood of these categories to the decision-maker.[18] In some cases, the information is published in scientific journals as proof of validity but in other cases, it may be kept completely confidential as part of the model’s trade secret.[19] There are several reasons why policymakers prefer transforming risk scores out of their probabilistic language: the risk categories are easily digestible by an uninformed audience, the results are pre-contextualized, and the decisions are more consistent with predetermined options. However, the transition can greatly obscure the true risk posed by the individual. The decision to develop an instrument with a five category ranking system, for example, may be decided before the data is even analyzed. Developers then have to manipulate the true output to fit into the arbitrary ranking system, resulting in an improper dispersion of risk ranges.[20]
To make matters worse, judges, defense attorneys, and prosecutors often have no method of interpreting the actual probability of the outcome in each category since each tool sets their own thresholds. In the same day, a judge may see the results of two separate tools labeling someone as high-risk of reoffending where one means there is an 8 percent chance and the other means there is a 58 percent chance. Often, the intuitive interpretation of the risk category greatly exaggerates the true risk posed. For example, the widely used PSA-Court tool labels defendants as high-risk with only an 8.6 percent chance of being re-arrested for a violent charge. [21] If decision-makers are not given the predicted probability, then triage must be solely based on the accused’s risk relative to others, rather than the public’s and decision-maker’s actual risk tolerance.
Decision Thresholds
In many cases, risk rankings are coupled with a recommendation based on a predetermined risk tolerance threshold. This transition point from prediction to recommendation is where the most important decisions are made and where instruments are most vulnerable to manipulation. For instance, low-risk individuals may be recommended for release, medium-risk may be recommended for low bail or programs, and high risk are recommended for detention. Thresholds may also be set to disqualify a defendant for a certain program or outcome; a developer could even tactfully redline some groups from certain risk thresholds.[22] This type of discrimination would be undetectable since the model would still appear well-calibrated if all the strata have an equal likelihood of the outcome.[23]
Threshold design may also introduce arbitrary, non-predictive factors beyond what well-validated actuarial instruments conclude. The YLS/CMI tool, for example, combines a young person’s risk score with their most serious arrest charge class to determine probation requirements, even though research shows that charge class has no correlation with risk of rearrest.[24] Tools like this that conflate validated risk scores for, for example, returning to court, with, for example, charge types are motivated by policymakers who need buy-in from local decision-makers.
Despite the multiple opportunities for policy decision to impact how tools are created, these instruments are packaged as data science, objective, unbiased and predictive. Defenders, whether at the table with developers or arguing against a bloated risk score in a court room, need to deepen their understanding of how these tools are developed and what decisions were made to produce them. We should question any system that attempts to automatically pre-determine rations of liberty and due process owed to any individual person accused of a crime.
The American Public Defenders’ Dilemma
Defenders of the accused, and the communities most often subjects of risk assessments, have different levels of access to the decisions that have been made within the development of any tool they confront in the courtroom and are rarely consulted during their development.[25]
Predictably, in jurisdictions that do consult defenders, their priorities and concerns around risk assessment instruments differ in some ways and align in others with the judges and prosecutors.
Consensus does not exist between defenders and other civil rights groups about whether RAI’s improve or worsen an unfair system. For defenders, their challenge in a triage system has been and continues to be slowing down the judge to deliberately and mindfully make a decision about the person standing at the podium. Both categorical and ordinal scores undermine that individuality and present a system where rations have already been divided. That said, too much time spent on the process in the pressurized environment of arraignments, for example, unnecessarily prolongs detention for some. Additional concerns defenders weigh regard fairness; is the accused being unfairly scored based on zip code, race, or socioeconomic status? Are the factors calculated against her in the tool failing to predict what she will do in the future based on some factor not measured? Some defenders have concluded that RAI’s improve fairness, protecting some people from randomly punitive or biased judges.[26] Others are more critical of how RAI’s are developed and implemented.[27] Considerations all advocates for justice should consider when weighing what role risk assessments should play include constitutional principles of liberty, due process and equal protection, as well as the limits of what risk assessment instruments help us understand about an individual as opposed to a system.
American Constitutional Framework for Analyzing Actuarial Decisions
Constitutional considerations frame defenders’ analysis. Most significantly, incorporating risk assessment instruments, whether they are assessing flight or dangerousness, imports a factor into any decision-maker’s analysis that may otherwise not exist: the probability of this person acting like other people who share certain measurable characteristics. Constitutional principles of due process guarantee individualized assessment of past crimes, not the probability of future decision-making or disparate punishment based on social, demographic or geographic affiliations.[28] A Wisconsin court already held that the weight of this probability should be considered minimal in such a system and never be the deciding factor in any case.[29]
In addition to the due process right of an individualized decision rather than judgment based on some grouping, how protected classes like race, ethnicity, religion, gender, age, socioeconomic status, and residential status are incorporated directly or indirectly into actuarial decision-making tools raise constitutional equal protection concerns. Some cities additionally have human rights laws preventing the implementation of tools that will have a disparate impact on any specifically protected population.[30] Even if tools remove explicit references to protected classes, this does not prevent them from having a disparate impact. Any factors that are commonly weighed, including one’s residential status, possession of a phone, prior arrest history, drug conviction history, family history, are indirectly related to protected factors. Moreover, if different class members have different prevalence rates of the outcome being predicted, then a valid prediction tool should predict disparate rates of the outcome by class regardless of whether it is a factor in the model.
Constitutional due process rights to confrontation and compulsory process in the criminal justice system also require increased transparency of assessment tools and how the risk scores they produce are calculated. Many tools are commercial: agencies or companies invoke trade secret protections in open record demands and in response to subpoenas.[31] The impact of access can be key: an expert can persuade a judge to suppress the tool’s recommendation from his sentencing decision.
A final constitutional consideration for defenders is whether the separation of powers is problematically imbalanced by risk assessment instruments commissioned by elected executive administrations. Some administrations may be more aligned with defenders’ principles of releasing people and guiding judges towards a threshold that limits incarceration. In jurisdictions discontinuing cash bail under public pressure, the introduction of risk assessment instruments has been closely tied to reducing reliance on incarceration.[32] However, other administrations may re-align the tools towards a desired outcome. For example, the Trump administration adjusted the risk of flight tool that the immigration enforcement agencies used to decide which people to arrest for deportation so that everyone would score a high risk requiring detention.[33]
Weighing Actuarial Decision-Making in the Criminal Justice System
Defenders know as well as any actor in the criminal justice system that in reality the judicial branch, despite principled aspirations of protecting constitutional rights and independence from politics, is greatly influenced by the public’s perception of its actions. New York City judges have long feared being on the cover of the tabloids and harangued for releasing someone who later commits a violent offense. Even as a large majority of elected officials, community groups and others have endeavored to close Rikers Island and massively reduce the number of people detained pretrial, New York City judges have continued to conservatively ration which people get released.[34]
In this context, it is easy to see why defenders may view risk assessment instruments that will encourage release as an advantage despite due process concerns: it gives judges some cover and often gives defenders a statistic rather than speculation to support their argument that someone will return to court. It also gives at least a superficial impression of neutral unbiased decision-making. If judges uniformly follow the recommendations of the tool and apply the weight of the tool similarly in all decisions, then it would appear they are treating everyone the same. Some defenders strongly prefer the fairness this promises over the trauma of watching an unfortunate group of people suffer at the whims of particularly punitive or biased judges.
Defenders’ inclination to protect clients generally from the most biased and most punitive decision-makers should not send them decisively on the side of actuarial tools. Research has already demonstrated how RAI decision-making tools built on biased training and testing data can amplify and camouflage biased decision-making across all decision-makers rather than ending it.[35] Advocates may have reasons for supporting some risk assessment instruments in the criminal justice system, but eliminating bias should not be one of them. Human bias will not be cured by replacing decision-makers with actuarial tools.
The public, as well as advocates in the justice system, should be involved in the decisions about what factors should and should not be considered in tools, where thresholds for recommendations are set, and how assessments are communicated, whether categorically or numerically.[36] Assessments should play a limited role in the criminal justice system: to assess how judges and other decision-makers are relying on racial bias more predictably than any other factor. Assessments can predict only one thing: how the system will ration justice. Not how any individual will behave in the future.
Julie Ciccolini is a Paralegal in the Special Litigation Unit of The Legal Aid Society where she conducts research and data science in support of the Society’s criminal justice reform efforts. In 2018, Julie Ciccolini received her Master’s in Human Rights from Columbia University. Her concentration focused on the human rights implications of using predictive and surveillance technology in the criminal justice system.
Cynthia Conti-Cook is a Staff Attorney with The Legal Aid Society in New York City. She works in the Special Litigation Unit, leading impact litigation and supporting defenders through training, consulting and research. In September 2018, she joined Data & Society Research Institute as a 2018-2019 fellow where she is focusing on expanding her work on engaging public defenders with data-driven practices.
Photo: Law scales on table. Symbol of justice | Shutterstock
References:
Administrative Office of the United States Courts, and Probation and Pretrial Services Office. “An Overview of the Federal Post Conviction Risk Assessment,” June 2018. http://www.uscourts.gov/sites/default/files/overview_of_the_post_conviction_risk_assessment_0.pdf.
Alper, Mariel, Ebony Ruhland, Edward Rhine, Kevin Reitz, and Cecelia Klingele. “Increasing Use of Risk Assessment at Release in The Continuing Leverage of Paroling Authorities: Findings from a National Survey.” Text, March 23, 2016. https://robinainstitute.umn.edu/publications/data-brief-increasing-use-risk-assessment-tools-release.
Corbett-Davies, Sam, Emma Pierson, Avi Feller, Sharad Goel, and Aziz Huq. “Algorithmic Decision Making and the Cost of Fairness,” 797–806. ACM Press, 2017. https://doi.org/10.1145/3097983.3098095.
Dwork, Cynthia, Moritz Hardt, Toniann Pitassi, Omer Reingold, and Rich Zemel. “Fairness Through Awareness.” ArXiv:1104.3913 [Cs], April 19, 2011. http://arxiv.org/abs/1104.3913.
Edwards, Ezekiel. “Predictive Policing Software Is More Accurate at Predicting Policing Than Predicting Crime.” American Civil Liberties Union (blog), August 31, 2016. https://www.aclu.org/blog/criminal-law-reform/reforming-police-practices/predictive-policing-software-more-accurate.
Eion Healy (2015). Research Report for MOCJ’s Pretrial Felony Re-Arrest Risk Assessment Tool. New York, NY: New York Cit,” n.d.
Fratello, Jennifer, Annie Salsich, and Sara Mogulescu. “Juvenile Detention Reform in New York City: Measuring Risk through Research.” New York: Vera Institute of Justice, Center on Youth Justice, April 2011. https://storage.googleapis.com/vera-web-assets/downloads/Publications/juvenile-detention-reform-in-new-york-city-measuring-risk-through-research/legacy_downloads/RAI-report-v7.pdf.
Garrett, Brandon L., and John Monahan. “Judging Risk.” Duke University School of Law, July 20, 2018, 1–43.
Gassert, Rachel. “Deep End Reform: The New York City Experience.” presented at the Deep End Inter-Site Conference, Chicago, Illinois, June 20, 2013. https://www.aecf.org/m/blogdoc/blog-newyorkcityexperience-deependreform.pdf.
Gouldin, Lauryn. “Disentangling Flight Risk from Dangerousness.” BYU Law Review 2016, no. 3 (April 30, 2016): 837–98.
Harcourt, Bernard E. “Risk as a Proxy for Race: The Dangers of Risk Assessment.” Federal Sentencing Reporter 27, no. 4 (April 2015): 237–43. https://doi.org/10.1525/fsr.2015.27.4.237.
Hardt, Moritz, Eric Price, and Nathan Srebro. “Equality of Opportunity in Supervised Learning.” ArXiv:1610.02413 [Cs], October 7, 2016. http://arxiv.org/abs/1610.02413.
Hoge, Robert D., D.A. Andrews, and Alan W. Leschied. “YLS/CMI,” n.d. http://www.fcro.nebraska.gov/pdf/yls-cmi-form.pdf.
James, Nathan. “Risk and Needs Assessment in the Federal Prison System.” Congressional Research Service, July 10, 2018. https://fas.org/sgp/crs/misc/R44087.pdf.
Laura and John Arnold Foundation. “Public Safety Assessment: Risk Factors And Formula,” n.d. https://www.arnoldfoundation.org/wp-content/uploads/PSA-Risk-Factors-and-Formula.pdf.
Levitt, Steven D. “The Relationship Between Crime Reporting and Police: Implications for the Use of Uniform Crime Reports.” Journal of Quantitative Criminology 14, no. 1 (1998): 61–81.
Maltz, Michael. “Bridging Gaps in Police Crime Data.” U.S. Department of Justice, September 1999. https://www.bjs.gov/content/pub/pdf/bgpcd.pdf.
Mayson, Sandra G. “Dangerous Defendants.” Yale LJ 127 (2017): 490.
Mitchell, Ojmarrh, and Michael S. Caudy. “Examining Racial Disparities in Drug Arrests.” Justice Quarterly 32, no. 2 (2015): 288.
“Level of Services Inventory-Revised Participant Manual,” n.d. http://dhs.sd.gov/drs/recorded_videos/training/lsi-r/doc/LSI-R%20introductory%20training%20Participant%20Manual.pdf.
“National Information on Offender Assessments, Part II.” Vera Institute of Justice, Center on Sentencing and Corrections, May 27, 2010. https://www2.illinois.gov/idoc/Documents/National_Information_Offender_Assessments_PartII_Memo.pdf.
Northpointe. “COMPAS Risk & Need Assessment System: Selected Questions Posed by Inquiring Agencies,” 2012. http://www.northpointeinc.com/files/downloads/FAQ_Document.pdf.
“Pretrial Risk Assessment Can Produce Race-Neutral Resultes.” Pretrial Justice Institute, 2017. https://university.pretrial.org/HigherLogic/System/DownloadDocumentFile.ashx?DocumentFileKey=5cebc2e7-dfa4-65b2-13cd-300b81a6ad7a.
Starr, Sonja B. “The New Profiling: Why Punishing Based on Poverty and Identity Is Unconstitutional and Wrong.” Federal Sentencing Reporter 27, no. 4 (April 1, 2015): 229–36. https://doi.org/10.1525/fsr.2015.27.4.229.
“The Use of Pretrial Risk Assessment Instruments: A Shared Statement of Civil Rights Concerns,” 2018. http://civilrightsdocs.info/pdf/criminal-justice/Pretrial-Risk-Assessment-Full.pdf.
Wexler, Rebecca. “Life, Liberty, and Trade Secrets: Intellectual Property in the Criminal Justice System.” SSRN Electronic Journal, 2017. https://doi.org/10.2139/ssrn.2920883.
Wilson, David B., Tammy Rinehart Kochel, and Stephen D. Mastrofski. “Race and the Likelihood of Arrest.” In Encyclopedia of Criminology and Criminal Justice, edited by Gerben Bruinsma and David Weisburd, 4245–51. Springer New York, 2014. https://doi.org/10.1007/978-1-4614-5690-2_245.
[1] Merriam-Webster, s.v. “triage,” accessed November 3, 2018, https://www.merriam-webster.com/dictionary/triage. Virginia Eubanks also talks about how algorithmic tools are used for economic rationing. https://www.nature.com/articles/scientificamerican1118-68
[2] Id. See also: https://www.merriam-webster.com/dictionary/try#h1 (“Middle English trien, from Anglo-French trier to select, sort, examine, determine, probably from Late Latin tritare to grind, frequentative of Latin terere to rub”)
[3] Alper et al., “Increasing Use of Risk Assessment at Release in The Continuing Leverage of Paroling Authorities: Findings from a National Survey,” Text, March 23, 2016, https://robinainstitute.umn.edu/publications/data-brief-increasing-use-risk-assessment-tools-release.
[4] “National Information on Offender Assessments, Part II” (Vera Institute of Justice, Center on Sentencing and Corrections, May 27, 2010), https://www2.illinois.gov/idoc/Documents/National_Information_Offender_Assessments_PartII_Memo.pdf.
[5] The factors that judges may lawfully consider in bail hearings vary depending on the statutes and judicial precedent in each state.
[6] “National Information on Offender Assessments, Part II.” Vera Institute of Justice, Center on Sentencing and Corrections, May 27, 2010. https://www2.illinois.gov/idoc/Documents/National_Information_Offender_Assessments_PartII_Memo.pdf.
[7] Steven D. Levitt, “The Relationship Between Crime Reporting and Police: Implications for the Use of Uniform Crime Reports.” Journal of Quantitative Criminology 14, no. 1 (1998): 61.; Michael D. Maltz, “Bridging Gaps in Police Crime Data” (U.S. Department of Justice, n.d.), https://www.bjs.gov/content/pub/pdf/bgpcd.pdf; Ojmarrh Mitchell and Michael S. Caudy, “Examining Racial Disparities in Drug Arrests.”Justice Quarterly 32, no. 2 (2015): 288; David B. Wilson, Tammy Rinehart Kochel, and Stephen D. Mastrofski, “Race and the Likelihood of Arrest.” In Encyclopedia of Criminology and Criminal Justice, edited by Gerben Bruinsma and David Weisburd, 4245. Springer New York, 2014
[8] Ezekial Edwards, “Predictive Policing Software Is More Accurate at Predicting Policing Than Predicting Crime.” American Civil Liberties Union. Accessed April 17, 2018. https://www.aclu.org/blog/criminal-law-reform/reforming-police-practices/predictive-policing-software-more-accurate.
[9] Measure of America, Social Science Research Council. 2018. DATA2GO.NYC, Accessed November 5, 2018, https://data2go.nyc/com/?id=107*36047015900*ahdi_puma!undefined!ns*!other_pop_cd_506~ahdi_puma_1~sch_enrol_cd_112~age_pyramid_male_85_plus_cd_20~median_household_income_puma_397~median_personal_earnings_puma_400~dis_y_perc_puma_102~poverty_ceo_cd_417~unemployment_cd_408~pre_k_cd_107!*air_qual_cd~major_felonies_per_1000_cd*family_homeless_cd_245#10/40.8276/-73.9588
[10] Sonja B. Starr, “The New Profiling: Why Punishing Based on Poverty and Identity Is Unconstitutional and Wrong,” Federal Sentencing Reporter 27, no. 4 (April 1, 2015): 229–36, https://doi.org/10.1525/fsr.2015.27.4.229.
[11] Bernard Harcourt, “Risk as a Proxy for Race: The Dangers of Risk Assessment.” Federal Sentencing Reporter 27, no. 4 (April 2015): 239. https://doi.org/10.1525/fsr.2015.27.4.237.
[12] Cynthia Dwork et al., “Fairness Through Awareness,” ArXiv:1104.3913 [Cs], April 19, 2011, 11, http://arxiv.org/abs/1104.3913.
[13] Brandon L. Garrett and John Monahan, “Judging Risk,” Duke University School of Law, July 20, 2018, 1–43.
[14] Nathan James, “Risk and Needs Assessment in the Federal Prison System” (Congressional Research Service, July 10, 2018), https://fas.org/sgp/crs/misc/R44087.pdf.
[15] Maria Cilenti & Elizabeth Kocienda, “Report on Legislation by The Criminal Courts Committee, The Criminal Justice Operations Committee, The Criminal Law Committee, and The Corrections and Community Reentry Committee” (New York City Bar, June 2015), https://www2.nycbar.org/pdf/report/uploads/20072469-SexOffenderRegistrationActReport.pdf.
[16] Ibid, pg. 2 & 4
[17] Models can be tailored to account for differences in the variables based on predictions of changes over time. However, for the most part, models assume the future will be exactly like the past.
[18] “Level of Services Inventory-Revised Participant Manual” (n.d.), http://dhs.sd.gov/drs/recorded_videos/training/lsi-r/doc/LSI-R%20introductory%20training%20Participant%20Manual.pdf.; Northpointe, “COMPAS Risk & Need Assessment System: Selected Questions Posed by Inquiring Agencies” (2012), http://www.northpointeinc.com/files/downloads/FAQ_Document.pdf.; Laura and John Arnold Foundation, “Public Safety Assessment: Risk Factors And Formula” (n.d.), https://www.arnoldfoundation.org/wp-content/uploads/PSA-Risk-Factors-and-Formula.pdf.; Robert D. Hoge, D.A. Andrews, and Alan W. Leschied, “YLS/CMI” (n.d.), http://www.fcro.nebraska.gov/pdf/yls-cmi-form.pdf.; Administrative Office of the United States Courts and Probation and Pretrial Services Office, “An Overview of the Federal Post Conviction Risk Assessment” (June 2018), http://www.uscourts.gov/sites/default/files/overview_of_the_post_conviction_risk_assessment_0.pdf.
[19] Rebecca Wexler, “Life, Liberty, and Trade Secrets: Intellectual Property in the Criminal Justice System,” SSRN Electronic Journal, 2017, https://doi.org/10.2139/ssrn.2920883.
[20] Eion Healy (2015). Research Report for MOCJ’s Pretrial Felony Re-Arrest Risk Assessment Tool. New York, NY: New York City Criminal Justice Agency. [unpublished]
[21] Sandra G. Mayson, “Dangerous Defendants,” Yale LJ 127 (2017): 490.
[22] Sam Corbett-Davies et al., “Algorithmic Decision Making and the Cost of Fairness” (ACM Press, 2017), 797–806, https://doi.org/10.1145/3097983.3098095; Moritz Hardt, Eric Price, and Nathan Srebro, “Equality of Opportunity in Supervised Learning,” ArXiv:1610.02413 [Cs], October 7, 2016, http://arxiv.org/abs/1610.02413.
[23] Ibid.
[24] Rachel Gassert, “Deep End Reform: The New York City Experience” (June 20, 2013), https://www.aecf.org/m/blogdoc/blog-newyorkcityexperience-deependreform.pdf.; Jennifer Fratello, Annie Salsich, and Sara Mogulescu, “Juvenile Detention Reform in New York City: Measuring Risk through Research” (New York: Vera Institute of Justice, Center on Youth Justice, April 2011), https://storage.googleapis.com/vera-web-assets/downloads/Publications/juvenile-detention-reform-in-new-york-city-measuring-risk-through-research/legacy_downloads/RAI-report-v7.pdf.
[25] Unlike judges and prosecutors, who are organized in local systems, defenders are less centrally and consistently organized. Some jurisdictions do not have defender offices and contract with individual lawyers to provide defense services. See https://www.nacdl.org/ResourceCenter.aspx?id=21260 for a list of defender offices across the country.
[26] https://university.pretrial.org/HigherLogic/System/DownloadDocumentFile.ashx?DocumentFileKey=5cebc2e7-dfa4-65b2-13cd-300b81a6ad7a at p. 6.
[27] http://civilrightsdocs.info/pdf/criminal-justice/Pretrial-Risk-Assessment-Full.pdf
[28] Starr, Sonja B. 2014. “Evidence-based sentencing and the scientific rationalization of discrimination.” Stan. L. Rev. 66:803. “Economists often defend statistical discrimination as efficient, arguing that if a decisionmaker lacks detailed information about an individual, relying on group-based averages… will produce better decisions in the aggregate. But the Supreme Court has held that this defense of gender and race discrimination offends a core value embodied by the Equal Protection Clause: people have a right to be treated as individuals”
[29] Loomis cautioned courts using risk assessments that they are only able to identify a group of high risk offenders and not a particular high risk individual, that “an offender who is young, unemployed, has an early age at first arrest and history of supervision failure, will score medium or high on the COMPAS Violence Risk Scale even though the offender never had a violent offense” (Wisconsin v. Eric Loomis, 2016).
[30] https://www1.nyc.gov/site/cchr/law/biased-based-profiling.page
[31] https://review.law.stanford.edu/wp-content/uploads/sites/3/2018/06/70-Stan.-L.-Rev.-1343.pdf
[32] http://www.abajournal.com/news/article/california_ends_financial_bail_in_favor_of_pretrial_risk_assessments
[33] http://www.abajournal.com/news/article/ice_risk_assessment_tool_now_only_recommends_detain/
[34] https://fivethirtyeight.com/features/youve-been-arrested-will-you-get-bail-can-you-pay-it-it-may-all-depend-on-your-judge/
[35] http://uk.businessinsider.com/amazon-built-ai-to-hire-people-discriminated-against-women-2018-10; https://hrdag.org/2016/10/10/predictive-policing-reinforces-police-bias/
[36] For a more complete set of principles, see Leadership Conference…
Published on November 8, 2018.